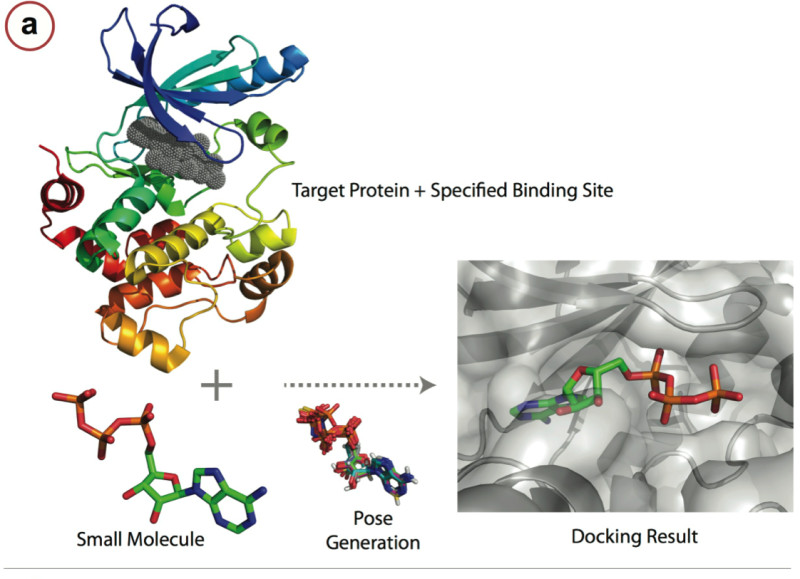
OIST researcher uses a computational approach in identifying proteins that will interact with potential drugs to speed up the process of drug discovery.
One of the major problems in today's society is the efficiency and cost of developing medicines to treat disease. The advancements in pharmaceutical science have been phenomenal, but the price of these advances remains prohibitively high for many pharmaceutical companies to venture into rare diseases. A large number of "neglected" diseases exist in which each disease has only a small number of patients in the world, yet the number is still significant. Kun-Yi Hsin, a researcher in the Open Biology Unit led by Prof. Hiroaki Kitano, is working on precisely this problem. In a recent article published inPLOS ONE, he describes his work identifying potential drugs and targets for those drugs using a computational approach that has the potential to bring the cost of drug development down while increasing the speed of drug discovery.
There are several approaches academic and pharmaceutical scientists use to determine whether a small molecule, which is the active component of a drug, interacts with a protein. Many diseases occur because a protein is over or under active. The goal for drug discovery is to find a small molecule that will either turn the errant protein on or off. Traditionally, this is done in a lab, manually testing each small molecule with the protein that is known to be causing disease. This method is expensive and time-consuming. Once computers came along, scientists were able to virtually identify candidate small molecules and then go on to test them in the lab. One of the problems with this approach is that the computational portion only gives you one piece of information, whether or not a small molecule binds to a specific protein. It cannot tell you about what other proteins with which the small molecule may be interacting. This becomes a problem when these unexpected interactions lead to unwanted consequences, or side effects. The results of unexpected side effects are increased risks in taking the drug for the patient, increased cost for the patient, and in extreme cases, withdrawal of a drug from the market.
This is where Hsin's research can help. He uses a method called systems docking simulation, which means he virtually assesses whether a small molecule will bind to a protein. However, instead of screening many small molecules and one misbehaving disease-causing protein, he screens one small molecule against many proteins. This results in him finding on- and off-targets, or the protein they want to change and proteins they may not want to change but that the small molecule interacts with unexpectedly. This allows the researchers to predict the toxicity of a given drug before it even reaches the experimental stage. By using a combination of docking simulation algorithms and machine learning systems, the researchers showed that their method was better able to predict on- and off-target candidates than the current methods in use.
The ultimate goal of his research is to use these computing methods to create cheaper drugs, leading to safer and more affordable medicine for patients. Right now Hsin says he is, "improving prediction results on a small scale before applying the methods to a bigger system." In the future, they have plans to test his system on the Influenza A Life Cycle pathway map (FluMap) in collaboration with the Institute of Medical Science, University of Tokyo. And moving forward, he will adapt his method with the computational power of the OIST High Performance Computing system (TOMBO) to more advanced prediction scenarios that more closely mimic the natural cellular environment.