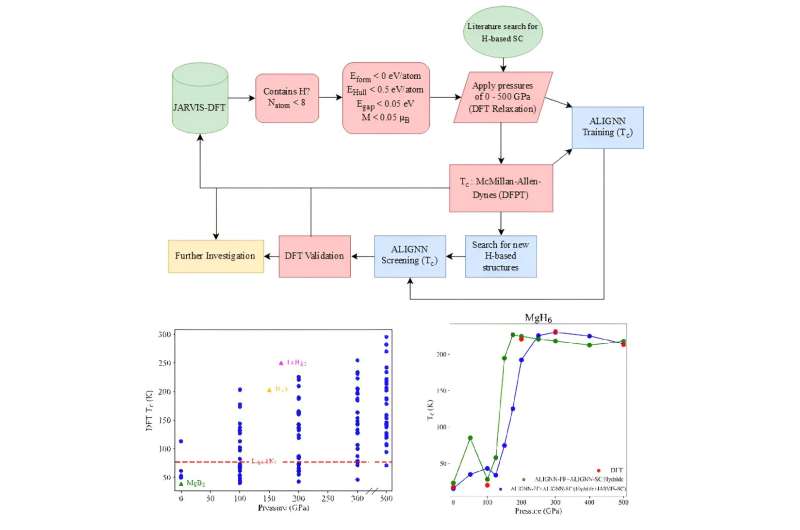
Superconductors are materials
that conduct electricity without resistance and are essential for several
technological advancements, which include medical imaging and energy-efficient
technology. However, most known superconductors operate under extreme conditions
such as extremely low temperatures or high pressures, which limit their
practical use.
Hydride compounds are a class of promising superconducting materials that possess high superconducting transition temperatures (i.e., H3S and LaH10) under ultrahigh pressures (several hundred GPa). Screening the entire space of potential new hydride-based materials that are superconducting at higher temperatures and under manageable conditions remains a significant challenge in physics and materials science.
Researchers Dr. Daniel Wines and Dr. Kamal Choudhary from the National Institute of Standards and Technology (NIST) have leveraged a combination of quantum mechanics-based density functional theory (DFT) and artificial intelligence (AI) to address this challenge. By integrating these methods, they developed an approach that enhances the prediction and discovery process for potential hydride superconductors.
The paper, titled "Data-driven Design of High Pressure Hydride Superconductors using DFT and Deep Learning," is published in the journal Materials Futures.
The researchers used high-throughput DFT calculations to predict the critical temperature of over 900 hydride materials under a range of pressures, finding over 120 structures with superior superconducting properties compared to MgB2, which has a critical temperature of 39 K.
To expedite the screening process and significantly reduce computational costs, they trained a graph neural network (GNN) model based on atomic structure that can instantly predict superconducting transition temperatures under various pressure conditions.
The data-driven approaches established by the NIST team provide a framework that can be used to screen new hydride superconductors in a faster and more efficient way. The integration of high-throughput quantum mechanical (DFT) simulations with machine learning can accelerate the prediction process prior to more costly experimental investigation.
Looking to the future, the field of high-pressure hydride superconductors is evolving rapidly. To continue advancing the field, it is critical to increase the volume of unique and high-quality data that is publicly available, which can in turn enhance the accuracy of machine learning models.
Wines states, "As researchers across the world continue to push the boundaries of materials science, the role of high-quality, publicly available datasets becomes more crucial. Relevant data can aid in refining our models and enhancing our predictive capabilities, which can lead to faster and more accurate discoveries."
The team at NIST encourages collaborative efforts and open sharing of data, which could be facilitated by platforms such as JARVIS (Joint Automated Repository for Various Integrated Simulations), which is an open access infrastructure hosted by NIST designed to automate materials discovery.
High temperature superconductors have the potential to revolutionize technologies across several industries. This work not only showcases the synergy of combining quantum mechanical simulations with AI, but also paves a path towards a future where the dream of a room-temperature superconductor may one day be realized.